We’re seeing machines that can process tons of data on the spot, without waiting for a connection to the cloud. This shift is happening thanks to the power of edge AI, which combines AI and edge computing.
The result?
Faster decisions, smoother operations, and less reliance on cloud servers. It’s making everything more efficient and opening doors to new possibilities.
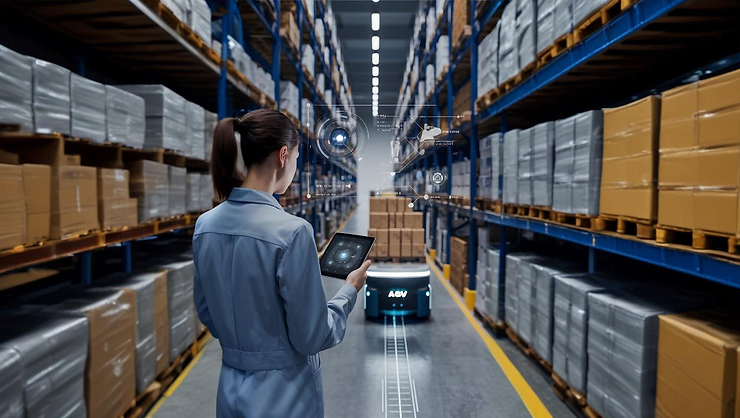
This article examines how Edge AI is assisting organizations in maintaining their competitiveness by transforming real-time data processing in autonomous systems.
Visit our Edge AI website to learn more about how we're improving products and services across various industries.
Key Role of Edge AI in Autonomous Systems
Autonomous systems require constant data from sensors, cameras, and other devices to make decisions. However, sending this data to centralized cloud servers introduces latency, which can impact the performance of time-sensitive applications such as self-driving vehicles or industrial robots. Edge AI solves this problem by processing data locally on devices or at the network edge, reducing latency and enabling real-time decision-making.
By offloading processing from the cloud to edge devices, businesses benefit from faster response times, greater control over data, and improved system robustness. These improvements are important in industries where even a millisecond of delay can impact safety or productivity. The implementation of Edge AI in autonomous systems thus provides businesses with a competitive edge, reducing costs, improving service reliability, and enhancing overall efficiency.
Hardware Considerations for Real-Time Edge AI Processing
For Edge AI to effectively power autonomous systems, the underlying hardware must be capable of real-time data processing. Key components include:
AI Accelerators: Devices such as Tensor Processing Units (TPUs), Neural Processing Units (NPUs), and Field-Programmable Gate Arrays (FPGAs) are optimized for accelerating AI computations, enabling Edge AI to process data with minimal delay.
High-Speed Processors: Processors like ARM Cortex-A or RISC-V offer the necessary computing power for Edge AI while ensuring energy efficiency, a major factor for battery-powered autonomous systems.
Memory and Bandwidth: Edge AI requires high-speed memory solutions (e.g., LPDDR5) and high-bandwidth interfaces (e.g., PCIe Gen4) to handle large volumes of data and transfer it rapidly between components.
Energy Efficiency: For autonomous systems like drones or robots, battery life is important. Edge AI solutions must be optimized for low power consumption, ensuring operational longevity without sacrificing performance.
These hardware components collectively ensure that Edge AI can meet the real-time demands of autonomous systems, offering businesses reliable and scalable solutions.
Software Solutions Supporting Edge AI for Real-Time Processing
Beyond hardware, software plays a major role in optimizing Edge AI for real-time processing:
Model Compression: Techniques like quantization, pruning, and distillation reduce AI model size, enabling faster processing on edge devices with limited resources.
Real-Time Operating Systems (RTOS): RTOS are designed for low-latency performance and deterministic task management, essential for time-critical applications in autonomous systems.
Edge AI Frameworks: Tools like ONNX, TensorRT, and OpenVINO optimize AI models for specific edge hardware, facilitating easier deployment across a variety of devices.
Federated Learning: This technique allows devices to collaboratively refine AI models locally, ensuring data privacy and scalability without needing to transfer sensitive data to the cloud.
These software solutions ensure that Edge AI delivers efficient and reliable real-time processing, empowering businesses to deploy autonomous systems at scale.
Connectivity for Real-Time Edge AI Data Processing
While Edge AI reduces dependence on cloud connectivity, reliable communication between devices or with the cloud is still necessary for certain scenarios:
5G Networks: 5G enables ultra-low latency and high data throughput, making it ideal for real-time communication in autonomous systems such as self-driving cars.
Wi-Fi 6 and LPWAN: These connectivity options offer high bandwidth and energy efficiency, making them suitable for industrial applications where edge devices need seamless communication.
Time-Sensitive Networking (TSN): In industrial automation, TSN ensures predictable and low-latency data transmission, enabling real-time control of autonomous robots and factory systems powered by Edge AI.
Efficient connectivity ensures that Edge AI can operate seamlessly across various environments, enhancing the performance of autonomous systems.
Real-World Applications of Edge AI in Autonomous Systems
Edge AI's practical benefits are evident in industries requiring real-time data processing. Key applications include:
Autonomous Vehicles: Edge AI supports real-time object detection, sensor fusion, and decision-making, ensuring the safety and efficiency of self-driving cars.
Robotics and Drones: Autonomous robots and drones rely on Edge AI for real-time navigation and task execution, reducing their dependence on cloud-based systems.
Healthcare: In healthcare, Edge AI makes it possible to track health data in real time and monitor patients continuously, enhancing response times and improving patient outcomes.
Industrial Automation: Edge AI powers predictive maintenance and anomaly detection in manufacturing, improving efficiency, reducing downtime, and cutting costs.
Visit our page to witness the creative results of our Vision Engineering experience in action.
Overcoming Challenges and Exploring Future Trends
Edge AI offers real-time processing but faces challenges like limited resources, energy efficiency, and data privacy, especially in sectors like healthcare and finance. Future innovations in neuromorphic computing, software frameworks, and edge-to-cloud synchronization will help businesses scale efficiently while reducing costs.
Integrating Edge AI into autonomous systems improves real-time data processing by reducing latency, easing bandwidth constraints, and lowering cloud reliance. For businesses aiming to stay competitive, adopting Edge AI is vital for discovering new opportunities and driving innovation.