Vision AI is redefining industries by enabling systems to employ machine learning and deep learning algorithms to evaluate and interpret visual data from video and image streams. Cloud-based infrastructure and Vision AI could be used to analyze and scale visual data at rates previously unheard of, improving operational efficiency and decision-making. This blog discusses how cloud computing enhances Vision AI performance and how Regami helps optimize Vision AI solutions for different sectors.
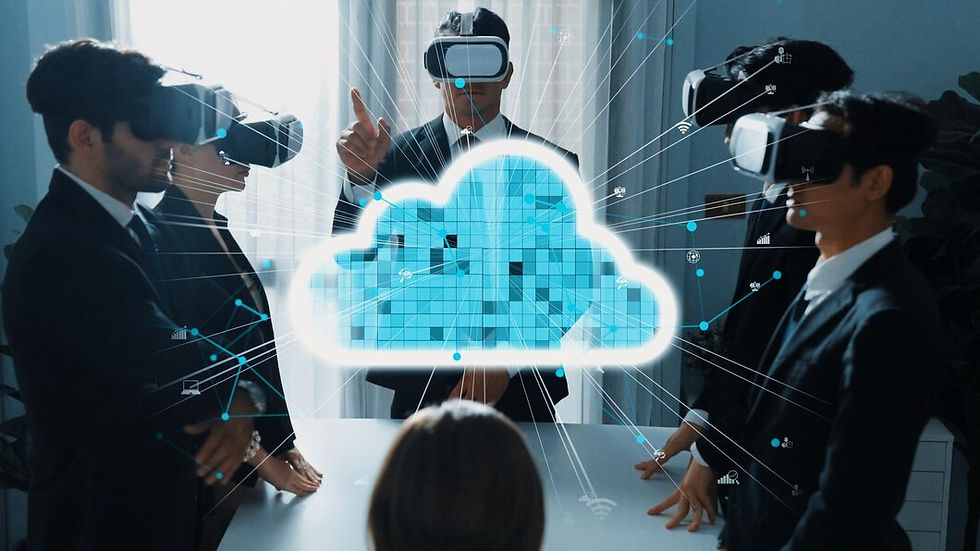
Are you looking to improve your cloud computing Vision AI performance? Examine our Cloud AI/ML solutions to see how Regami can use modern Vision AI technology to help your company grow and thrive.
The Power of Vision AI in the Cloud
Scalable, adaptable, and potent AI solutions are now made possible via the cloud. The cloud enables deep learning model training, large-scale data processing, and quicker decision-making when combined with Vision AI. Instead of depending on costly and constrained on-premises hardware, cloud systems provide almost limitless scalability and processing power. Without being limited by local computing, Vision AI systems will be able to process massive video feeds, evaluate high-resolution images, and offer real-time insights.
Cloud-based vision AI systems have a few significant benefits such as:
Scalability: Companies may use cloud infrastructure to scale their Vision AI solutions according to the volume of data they need to process, which makes managing varying workloads simpler.
Flexibility: The cloud gives companies the freedom to select the ideal mix of processing, storage, and processing power to meet their unique Vision AI requirements.
Real-Time Analysis: Whether for security, healthcare, or driverless cars, cloud-powered vision AI can instantly analyze photos and video streams to make decisions.
Key Challenges in Optimizing Vision AI Performance in the Cloud
While cloud infrastructure offers incredible benefits for Vision AI, there are certain challenges that need to be addressed to fully optimize performance. These challenges typically revolve around latency, data throughput, and computational demands.
1. Real-time processing and latency:
Assuring minimal latency is one of the main issues with cloud-based vision AI. Real-time image and video processing is essential in many use cases, including robots, autonomous cars, and security surveillance. Businesses must use edge computing, where data is first processed locally before being sent to the cloud for further analysis, and optimize their cloud infrastructures to reduce latency.
2. Bandwidth and Data Throughput:
Processing vast amounts of high-resolution photos or video streams is a common task for vision AI. If not properly managed, the sheer volume of data generated can strain bandwidth and impede performance.
3. Demands on Computation:
Vision AI's deep learning algorithms demand a lot of processing power, particularly when it comes to model training. The cloud provides scalable computing capacity.
Strategies for Optimizing Vision AI Performance in the Cloud
To address these challenges, businesses can implement the following strategies to enhance the performance of Vision AI systems in the cloud:
1. Leverage Edge Computing:
One effective approach to reducing latency and improving real-time performance is integrating edge computing with cloud-based Vision AI systems. Edge computing involves performing some level of data processing at the edge of the network (closer to where the data is generated), such as on local devices or servers.
2. Optimize Cloud Architecture for Throughput:
To ensure Vision AI systems can process large amounts of data quickly, businesses should optimize their cloud architecture for high throughput. This means selecting cloud services that offer high-speed data transfer and utilizing content delivery networks (CDNs) to distribute data efficiently.
3. Use GPU and Specialized Hardware for AI Workloads:
Vision AI relies heavily on deep learning models that benefit from the parallel processing capabilities of graphical processing units (GPUs). Many cloud platforms offer GPU-based instances designed to accelerate AI and machine learning workloads.
Regami's Contribution to Optimizing Vision AI in the Cloud.
At Regami, we specialize in helping businesses optimize Vision AI performance in the cloud, providing tailored solutions that meet the specific needs of each industry. Here's how we contribute:
Custom Cloud-Based Vision AI Solutions:
Regami designs and develops custom cloud-based Vision AI solutions that meet the unique needs of each client. Our solutions are built to handle large-scale data processing, real-time analysis, and advanced image recognition while ensuring the optimal performance of cloud infrastructure.
Edge Computing Integration:
Regami integrates edge computing with cloud-based Vision AI systems to minimize latency and ensure real-time decision-making. Our solutions ensure that visual data is processed locally, when necessary, while still benefiting from the power and scalability of the cloud.
Ready to use the cloud to boost your Vision AI capabilities? Examine our Vision Engineering options to see how Regami's experience can help you maximize the capabilities of your Vision AI.
Building Scalable, High-performance Vision AI Solutions in the Cloud
In conclusion, with enhanced speed, scalability, and efficiency, cloud-powered Vision AI is shifting industries. Businesses can exploit its potential by addressing issues like latency and data speed. By optimizing Vision AI technologies, Regami helps businesses maintain their edge in a data-driven environment.