AI-driven vision systems are driving innovation across sectors like robotics, smart cities, and manufacturing. These systems rely on advanced hardware to handle complex AI algorithms and real-time data processing. This blog focuses on the essential role of hardware engineering in supporting AI integration within vision systems.
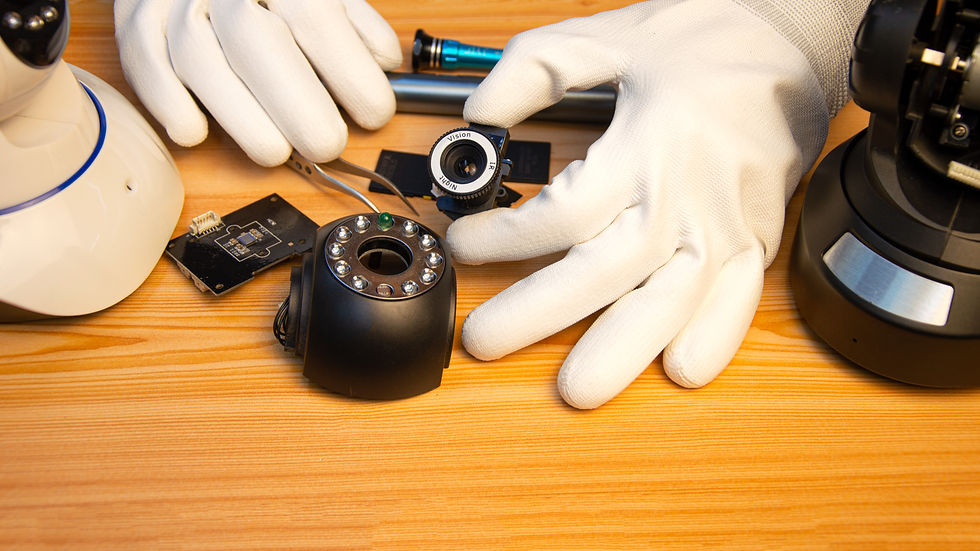
Uncover the impact of Regami’s hardware engineering solutions on innovation and success. To learn more and see our success stories, visit our Device engineering page.
The Role of Hardware in AI-Driven Vision Systems
Vision systems powered by AI require the seamless integration of multiple components, including sensors, processors, and memory units. Unlike traditional computing systems, these setups must support high-speed data acquisition, real-time processing, and energy efficiency. Achieving this balance is where hardware engineering excels.
Hardware engineers design custom systems to meet specific application needs. For instance, autonomous vehicles require cameras and LiDAR sensors to process data in milliseconds, demanding optimized designs with low latency and high throughput. Similarly, in industrial automation, vision systems must operate reliably in harsh conditions, requiring rugged and durable hardware.
Sensor Selection and Integration
One of the key elements in hardware engineering for vision systems is the selection and integration of sensors. The hardware must support a variety of sensor types, including cameras, depth sensors, and infrared sensors, all of which have unique data processing requirements. Engineers must ensure that these sensors communicate effectively with the processing units, minimizing data loss and ensuring high-quality image output.
For instance, integrating high-definition cameras with AI processors to recognize objects or people requires custom hardware that can handle large video streams at high frame rates. This demands not only efficient data transfer protocols but also the right choice of memory and processing units.
Key Challenges in Hardware Engineering for Vision Systems
Power Efficiency AI workloads are computationally intensive, often requiring specialized hardware like GPUs, TPUs, or ASICs. Designing hardware that delivers high performance while maintaining energy efficiency is a critical challenge. This is particularly vital for mobile and edge devices, where battery life is a significant constraint.
Thermal Management Vision systems generate considerable heat, especially during AI inference tasks. Effective thermal management solutions, such as advanced cooling systems or heat sinks, are essential to prevent overheating and ensure reliable operation.
Scalability As AI models become more complex, the hardware must scale to accommodate increased processing demands. This includes designing systems that can support future upgrades without significant overhauls.
Real-Time Performance Vision systems often require real-time data processing to deliver actionable insights. Hardware designs must minimize latency, ensuring that data flows seamlessly from sensors to processing units.
Security and Data Privacy With AI’s expanding role in sensitive applications like healthcare, ensuring secure hardware becomes paramount. From encryption to secure boot processes, hardware engineering must also prioritize privacy and cybersecurity concerns to protect sensitive data.
AI-Specific Hardware Considerations
Integrating AI into vision systems has led to the development of ASICs and SoCs, optimized for AI workloads, offering superior performance over general-purpose hardware. Examples include Google’s TPU and NVIDIA’s Jetson modules. Engineers are also incorporating NPUs into vision systems to accelerate AI tasks like object detection, face recognition, and natural language processing.
The Impact of Hardware Engineering on Vision Systems
Effective hardware engineering enhances the performance, reliability, and scalability of vision systems. Key areas of impact include:
Data Throughput
High-speed interfaces like PCIe and MIPI ensure rapid data transfer between sensors and processors, a necessity for high-resolution imaging applications.
Miniaturization
Advances in hardware engineering have enabled the miniaturization of components, making it possible to develop compact vision systems for wearable devices and drones. This enables the creation of vision systems that are lightweight yet powerful, ideal for applications such as augmented reality (AR).
Custom Designs
Custom hardware solutions tailored to specific AI workloads offer significant performance improvements over off-the-shelf components. For example, designing custom chips for image recognition or object tracking can dramatically increase processing speeds and reduce power consumption.
System Integration
The synergy between hardware and software is critical for vision systems. Engineers ensure that the hardware architecture supports the software’s needs, minimizing latency and maximizing the real-time performance of the system.
Emerging Trends in Hardware Engineering for AI Vision
Edge Computing
The shift toward edge computing places significant demands on hardware. Edge devices must process AI tasks locally, requiring powerful yet compact designs. Hardware engineers are innovating with SoCs and neuromorphic chips to meet these demands.
3D Integration
3D integrated circuits (3D ICs) stack multiple layers of components, reducing footprint and improving performance. This trend is particularly promising for compact vision systems, where space is a premium, but performance cannot be compromised.
FPGA-Based Solutions
Field-programmable gate arrays (FPGAs) offer flexibility and high performance for AI workloads, making them a popular choice for hardware prototyping and development. Their reconfigurability allows hardware engineers to quickly adjust designs to meet changing demands.
Advanced Sensor Integration
Modern vision systems leverage a variety of sensors, including infrared, ultrasonic, and hyperspectral cameras. Integrating these sensors into a unified hardware platform is a growing focus in hardware engineering. This trend allows vision systems to collect richer data, improving accuracy and enabling new capabilities in fields like medical diagnostics and environmental monitoring.
Quantum Computing
While still in its early stages, quantum computing is beginning to show promise for revolutionizing AI and vision systems. Hardware engineers are exploring quantum algorithms and quantum hardware to accelerate AI processes like image classification and object detection.
Utilize our hardware engineering expertise to create high-performance solutions that enhance your AI-powered vision systems. Explore our Vision engineering services today.
Future-Proofing AI Vision through Hardware Engineering
AI integration in vision systems has elevated hardware engineering, addressing power, thermal, and performance challenges. As AI evolves, hardware engineering will drive industry breakthroughs. Collaboration among engineers and developers is key to unlocking vision system potential, enabling smarter, more efficient solutions for complex challenges.