Camera Engineering
Ultra Low Latency Cameras For Autonomous Vehicle Navigation
Client Background:
Our client is a leading technology company specializing in autonomous vehicle navigation systems. Renowned for developing advanced self-driving vehicle solutions, the company focuses on enhancing safety and performance through cutting-edge sensors and artificial intelligence. Despite significant progress in the autonomous vehicle industry, the client faced a critical issue with camera latency, which hindered the real-time decision-making capabilities of their navigation systems. The latency prevented the vehicles from processing visual data quickly enough to adapt to rapidly changing environments.
Recognizing the importance of ultra-low latency for optimal performance, the company approached us to refine their camera systems and meet these stringent requirements.
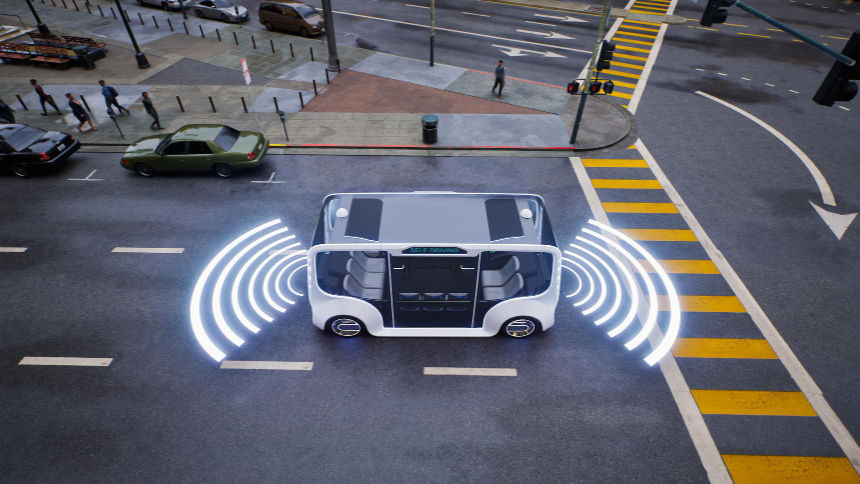
Challenges:
The client struggled with latency issues in their camera systems, which impacted the vehicle’s ability to process visual data in real time. As autonomous vehicles rely heavily on cameras for perception, these delays affected key functions such as collision avoidance and navigation accuracy. Slow processing times posed significant safety risks, particularly in fast-moving and unpredictable environments.
To ensure timely decision-making, the company needed a solution to reduce latency and enhance the overall performance of their autonomous vehicle systems.
Our Solutions:
To address the challenges faced by the client, we implemented several advanced solutions:
Advanced Image Processing: We deployed specialized algorithms designed to process images quickly, reducing the time taken to analyze visual data. This allowed the vehicle’s system to make faster decisions, improving obstacle detection and overall navigation.
High-Performance Camera Integration: Regami Solutions integrated ultra-low latency cameras capable of capturing clearer images at faster frame rates. This enhanced the vehicle’s ability to gather and interpret visual information quickly, supporting rapid decision-making even in challenging conditions.
Edge Computing Solutions: To minimize transmission delays, we implemented edge computing, processing data directly on the vehicle instead of sending it to remote servers. This solution significantly improved the vehicle's real-time response time.
Optimization for Real-Time Data: We optimized the network architecture, facilitating high-speed data transfer between the cameras and the vehicle’s central processing unit. This reduced bottlenecks, improving communication between sensors and the processing unit.
AI-Enhanced Visual Recognition: To further improve performance, we integrated AI-driven image recognition systems that quickly identified and classified objects. This enabled prompt, data-driven decisions, allowing the vehicle to adapt rapidly to environmental changes.
Robust Testing and Calibration: We conducted thorough testing and calibration of the camera and sensor systems to ensure they performed consistently under real-world conditions. This evaluation confirmed that our solution could handle environmental challenges while maintaining reliable performance.
Outcomes:
The outcomes of implementing our solutions were significant:
Reduction in Camera Delay: Our ultra-fast processing solutions dramatically reduced the visual data processing time, enabling near-instant decision-making. This enhancement improved both the safety and efficiency of the vehicle.
Enhanced Navigation Precision: With faster image processing and advanced recognition, the vehicle's navigation system became more accurate in assessing its surroundings. This led to better obstacle detection and improved route planning in dynamic environments.
Improved Real-Time Reaction: The vehicle’s camera system could now quickly adapt to evolving conditions, significantly enhancing its responsiveness. This improvement contributed to safer autonomous driving in urban and rural environments.
Reduced Collision Risk: By reducing delay and boosting processing speed, the vehicle could more effectively avoid potential accidents. This improved real-time response allowed for quicker adjustments or stops when necessary, reducing collision risk.
Boosted Customer Trust: The upgrades to the navigation system enhanced the client's reputation as a leader in autonomous vehicle safety and performance, building trust among customers and partners.
Cost Savings in Development and Deployment: By optimizing the camera systems and leveraging edge computing, the client reduced reliance on cloud computing and external server infrastructure, resulting in cost savings. These resources were then reallocated to further innovation.