Cloud AI/ML
Streamlining AI Model Deployment for Healthcare
Client Background:
The client is a leading healthcare organization with multiple branches, specializing in diagnostic imaging services. To improve patient outcomes and increase diagnosis accuracy, they make use of advanced artificial intelligence (AI). Significant delays prevented the organization from using AI models for its imaging systems, which led to inefficiencies and lost chances for better healthcare services. To increase model accuracy and expedite their AI deployment process, they need an experienced partner.
Regami stood out due to its proficiency in implementing AI and providing cloud solutions specifically designed for the healthcare sector.
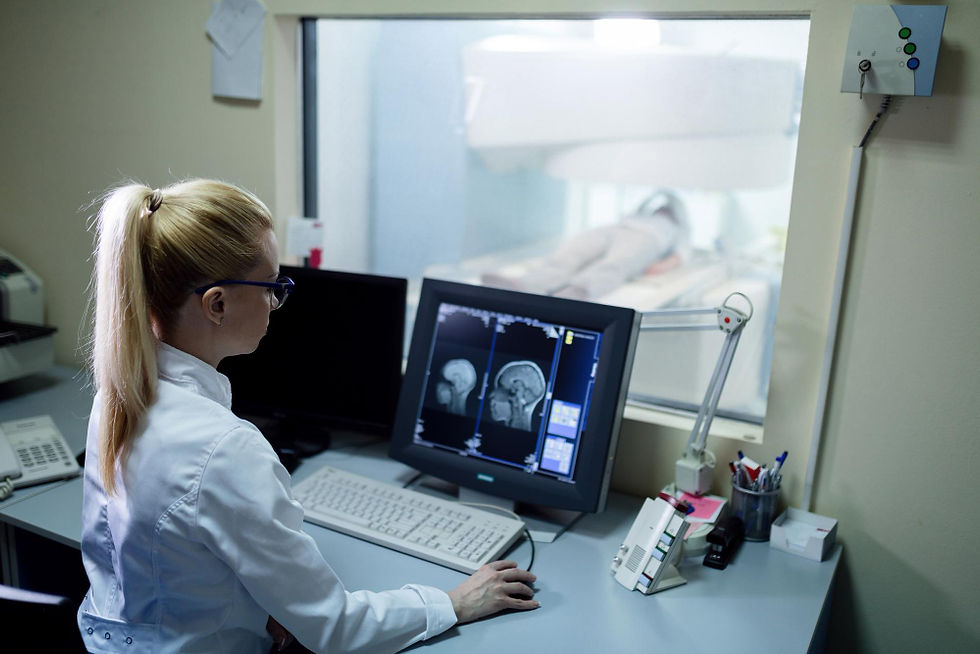
Challenges:
The healthcare company was having trouble implementing AI models in its diagnostic imaging systems for many reasons. Their current procedures were unscalable, slow, and prone to errors, which led to prolonged waiting periods for model updates and deployments. This made it more difficult for them to quickly incorporate advanced AI technologies, which had an impact on both diagnostic performance and operational efficiency.
To increase accuracy, accelerate deployment, and ensure the models could be quickly updated in a cloud environment, the customer requested assistance. They need a strong solution that would guarantee dependability, cut down on delays, and grow with their demands.
Our Solutions:
Our approach focused on leveraging advanced cloud infrastructure, automation, and continuous monitoring to streamline the entire deployment process while ensuring high standards of accuracy and security.
Cloud-Based Deployment: Regami transferred AI models to the cloud, offering a scalable and flexible platform for faster deployment and real-time updates, ensuring timely diagnostic insights.
Automated Model Monitoring: We introduced continuous monitoring to track model performance, ensuring quick identification of issues and minimizing downtime to keep the AI models accurate and reliable.
CI/CD Pipeline for Model Updates: A CI/CD pipeline automated model versioning and deployment, reducing update cycles from weeks to hours and enabling faster integration of new AI advancements.
Scalable Infrastructure: The cloud infrastructure was designed to scale with growing diagnostic data, ensuring no performance issues and future-proofing the client’s AI deployment.
Enhanced Data Security: We integrated encryption and compliance measures to ensure the solution met healthcare regulations and safeguarded patient data against unauthorized access.
Collaborative Workflow Integration: Regami worked closely with the client’s team to ensure smooth AI model integration into clinical workflows, promoting higher adoption and improving operational efficiency.
Outcomes:
The deployment of Regami’s solution resulted in significant improvements across several critical areas of the client’s operations. Here’s how our solution made a difference:
Reduced Deployment Time: Cloud infrastructure and CI/CD pipelines enabled faster deployment of AI models, allowing the client to integrate updates in hours rather than weeks, ensuring quick access to the latest AI advancements.
Improved Model Accuracy: Continuous monitoring and updates enhanced model consistency and precision, leading to more reliable diagnostic results and better decision-making for patient care.
Increased Scalability: The cloud-based solution allowed seamless scaling of AI models to handle growing data volumes without performance issues, ensuring future growth and innovation.
Enhanced Operational Efficiency: Automation of tasks like model monitoring and updates reduced manual intervention, minimized errors, and improved overall productivity for both medical and IT teams.
Stronger Security and Regulatory Compliance: The solution ensured compliance with healthcare regulations, securing patient data with encrypted storage and transmission and safeguarding against potential breaches.
Improved Collaboration Between Teams: A collaborative deployment process fostered better communication among teams, improving integration and ensuring smoother adoption of AI models in clinical workflows.