Cloud AI/ML
Reducing Cloud AI Costs for Retail
Client Background:
We partnered with a major retail chain to optimize their cloud-based AI and ML infrastructure, reducing operational costs while ensuring secure performance and scalability. Our client, a well-established retail chain operating hundreds of stores nationwide. Known for their commitment to customer-centric services, their company has integrated AI and machine learning into various aspects of its operations, from inventory management to personalized marketing, they found it difficult to strike the right balance between cost efficiency and maintaining the high-performance standards required for their AI models.
They reached out to Regami, in search of a solution that would reduce these escalating costs without compromising the quality or scalability of their AI applications.
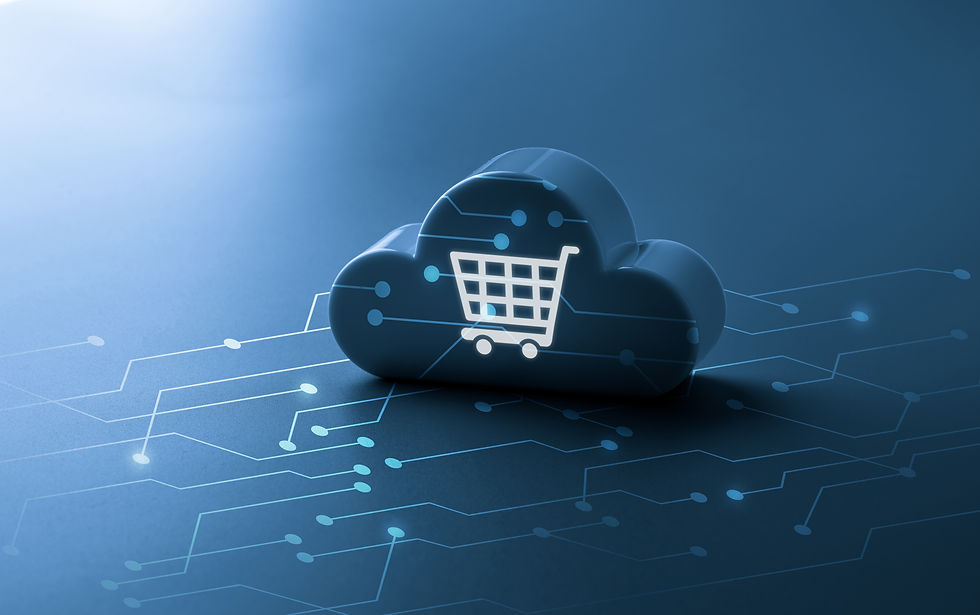
Challenges:
Controlling the expenses associated with their growing AI operations presented major challenges for our client's company. Their AI-powered apps demanded a lot of processing power, which caused the cost of cloud infrastructure to soar. Due to the outdated resource allocation approach, cloud resources have been frequently over-provisioned for unnecessary tasks, resulting in unnecessary expenditures. While performance has a direct influence on customer experience and operational efficiency, the organization was hesitant to compromise the accuracy and speed of their AI models.
They need an approach that would preserve optimal performance while streamlining AI workloads, cutting expenses, and optimizing their cloud infrastructure. We were tasked with bringing a solution that could directly address these issues.
Our Solutions:
Regami Solutions implemented a customized approach designed to streamline the client’s cloud infrastructure, reduce waste, and optimize their AI workflows while maintaining high performance.
Cloud Resource Optimization: We began by conducting a comprehensive audit of the client’s cloud architecture. Through this assessment, we identified areas of resource waste, including over-provisioned compute and storage resources. By rightsizing their infrastructure, we significantly reduced costs without impacting the performance of their AI workloads.
Dynamic Auto-Scaling for AI Workloads: To handle fluctuating demands, we implemented a dynamic auto-scaling solution that automatically adjusts cloud resources based on the specific needs of AI models. This ensured that the client only used and paid for the resources they required at any given time, optimizing cloud spend.
Serverless Computing for Machine Learning Tasks: For certain AI operations, we shifted to serverless computing, which allowed the client to pay only for the compute time used. This solution removed the need for dedicated servers to run continuously and resulted in substantial savings without performance loss.
Real-Time Cost Monitoring & Alerts: We introduced a comprehensive cost monitoring system with real-time analytics and automated alerts, helping the client identify and react to cost anomalies quickly. This proactive approach empowered them to make data-driven decisions and stay within budget.
Efficient Model Design and Deployment: We worked closely with the client’s AI team to optimize their machine-learning models. By refining algorithms and reducing computational complexity, we lowered the resource consumption required for training and inference, which directly reduced cloud infrastructure costs.
Implementing Cloud-Native Solutions: To improve cost efficiency, we migrated certain AI workloads to cloud-native platforms that were better suited for their needs. This shift resulted in enhanced performance and more cost-effective operations, making the overall cloud strategy more efficient.
Outcomes:
Our client was able to achieve real results that not only cut costs but also improved the efficiency and scalability of their AI operations.
Reduction in Cloud Costs: The optimization of cloud resources resulted in a substantial reduction in monthly operational costs while maintaining AI performance.
Reduction in Resource Wastage: Through dynamic auto-scaling and resource rightsizing, the client reduced unnecessary resource usage, ensuring that compute and storage were aligned with actual demand, eliminating idle capacity.
Enhanced Scalability with Cost Efficiency: The auto-scaling mechanism enabled the client to handle varying workloads seamlessly, scaling up during peak usage periods while scaling down during off-peak times, without overspending.
Improved AI Model Efficiency: By improving machine learning algorithms and reducing computational complexity, the client experienced improved model performance. The models now ran faster and with less computational overhead, leading to more efficient use of cloud resources.
Greater Cost Visibility and Control: With immediate cost tracking and alerts, the client had better visibility into their cloud expenses. This enabled them to make timely adjustments, preventing unexpected cost overruns and improving financial control.
Faster Deployment and Time to Market: Enhanced cloud infrastructure and streamlined AI processes allowed the client to reduce the time needed to deploy new AI-driven initiatives. This accelerated time-to-market for their technology-driven projects and enhanced their competitive advantage in the retail space.