Artificial Intelligence
Real-Time Data Processing in Smart Cities
Client Background:
The client is a leading technology provider specializing in smart city solutions. They work with municipal authorities to enhance urban infrastructure and improve the quality of life for residents. The client focuses on implementing solutions, including AI and IoT, to create more sustainable and efficient cities. With a growing urban population, the demand for smarter and more efficient city systems has increased. The client aims to streamline city operations, reduce energy consumption, and optimize public services.
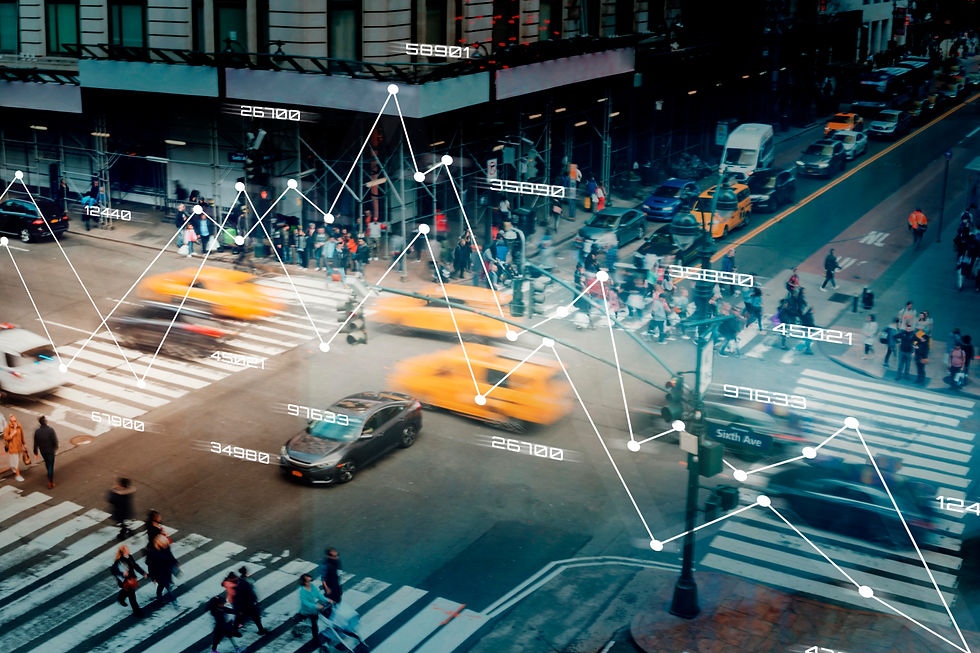
Challenges:
Real-time AI processing requires significant computational resources, straining existing infrastructure. The growing volume of data from sensors, cameras, and IoT devices in smart cities demands immediate analysis to deliver actionable insights. Traditional processing methods were insufficient to handle the scale and complexity of this data in real-time. The challenge was to ensure that the system could process this data instantly while maintaining performance, scalability, and minimal downtime. The infrastructure had to evolve to meet these needs without compromising efficiency.
Our Solutions:
We developed a real-time and consistent data processing system using AI and edge computing, enabling efficient and immediate processing of large-scale data.
Robust Edge AI Integration: Edge AI was integrated to handle data closer to the source, reducing latency and offloading computational power from central servers. This integration allowed for more responsive decision-making at the edge, ensuring faster data processing with minimal delays.
Optimized Traffic Management: We used AI to analyze traffic flow in real-time, optimizing signal timings and reducing congestion, improving city mobility. The system also provided insights into future traffic trends, allowing for proactive measures to further optimize traffic management.
Energy Consumption Analysis: AI-based data processing helped analyze and predict energy consumption patterns, enabling more efficient energy distribution and reducing waste. The system continuously adjusted energy use based on real-time data, further enhancing energy efficiency.
Predictive Maintenance: The system provided real-time insights into the condition of city infrastructure, allowing for predictive maintenance to avoid costly breakdowns. By forecasting potential failures, the system enabled timely interventions and minimized disruption to services.
Improved Public Safety: Continuous processing of surveillance and sensor data helped improve security, alerting authorities to incidents faster and optimizing emergency response times. The system also identified potential threats before they escalated, enhancing proactive public safety measures.
Outcomes:
The client successfully transformed their smart city infrastructure, providing real-time insights that enhanced urban living.
Increased Efficiency: Traffic congestion decreased, energy consumption was optimized, and city services became more efficient, improving overall city operations. This resulted in reduced delays in public services and smoother traffic flow across urban areas.
Sustainability Gains: The energy usage analysis helped reduce the city's carbon footprint by optimizing energy distribution. As a result, the city saw a measurable reduction in greenhouse gas emissions, contributing to a greener environment.
Enhanced Public Safety: Real-time data improved emergency response, reducing crime rates and increasing public safety. The proactive alerts provided by the system helped authorities act quickly, minimizing the impact of incidents on the public.
Cost Savings: Predictive maintenance minimized the need for expensive repairs and reduced downtime in critical infrastructure systems. By anticipating potential failures, the city cut emergency repair costs and prevented disruptions to services.
Adaptable for Future Growth: The Solution guaranteed that the system will address the growing data needs of cities in the future and provided options for scaling up. As the city expands, it is easier to adapt the system with the addition of new devices and sensors, without compromising the performance of existing sensors.