Camera Engineering
Pedestrian Monitoring with Edge AI-Enabled Cameras
Client Background:
Our client is a pioneer in urban transformation, leveraging cutting-edge technology to enhance public safety, optimize traffic flow, and create more sustainable environments. Their latest project focused on pedestrian monitoring to mitigate accidents, manage foot traffic efficiently, and improve overall safety in urban spaces.
To achieve their ambitious goals, they partnered with Regami to integrate advanced Edge AI solutions capable of delivering real-time insights and driving smarter urban management.
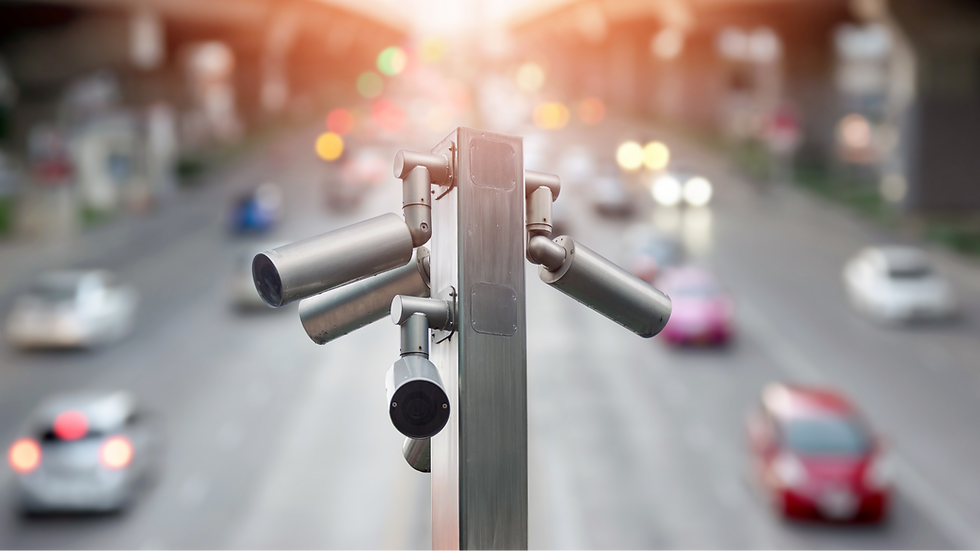
Challenges:
The client faced several challenges in implementing an effective pedestrian monitoring system as part of their smart city initiative. High-traffic areas were prone to frequent pedestrian-related accidents, and existing surveillance systems struggled to provide real-time tracking and behavioral analysis. This lack of immediacy often delayed critical interventions during emergencies. Additionally, the existing AI models were cloud-dependent, causing latency issues that further hindered responsive decision-making. Differentiating between pedestrians, vehicles, and obstacles in crowded or dynamic environments posed another significant challenge.
To address these limitations, the client required a robust, scalable solution that could ensure real-time tracking, enhance safety, and support the city's growing infrastructure needs.
Our Solutions:
Our team collaborated closely with the client to design and implement a tailored solution using edge AI-enabled cameras for advanced pedestrian monitoring:
Real-Time Pedestrian Tracking and Behavior Analysis: We deployed AI-powered edge devices equipped to detect and analyze pedestrian activity in real time. This enabled accurate monitoring of pedestrian movements and behaviors, ensuring quick identification of potential safety risks and enabling timely interventions.
Deep Learning Integration for Enhanced Detection: By integrating advanced deep learning algorithms, we improved the system’s ability to detect, classify, and predict the movement of pedestrians, vehicles, and other objects, even in highly crowded or low-visibility conditions.
On-Site Data Processing to Reduce Latency: The use of Edge AI allowed data to be processed locally on devices, eliminating the need for constant cloud communication. This reduced latency significantly, ensuring near-instantaneous responses during critical events.
Scalable Architecture for Future Growth: The solution was built on a modular and scalable architecture, enabling seamless expansion as the city’s infrastructure grew. This adaptability ensured future compatibility without requiring extensive system overhauls.
Secure Data Transmission and Privacy Measures: To address concerns regarding data security and privacy, we implemented end-to-end encryption and secure storage protocols. These measures ensured compliance with privacy regulations while fostering public trust in the system.
Integration with Smart City Infrastructure: The solution was designed to integrate effortlessly with existing smart city infrastructure, such as traffic management systems and emergency response networks, creating a unified ecosystem for urban management.
Outcomes:
The implementation of our pedestrian monitoring solution delivered substantial benefits, transforming urban safety and operational efficiency for the client.
Enhanced Pedestrian Safety: Real-time detection and behavioral analysis significantly reduced accidents by enabling immediate interventions in hazardous situations. Pedestrian zones became safer, with reduced risks of collisions and incidents.
Optimized Traffic Flow: The system’s ability to monitor and manage pedestrian movement improved overall traffic management. It enabled better coordination between foot and vehicular traffic, alleviating congestion in busy areas.
Reduced Response Time in Emergencies: The low-latency processing capabilities of Edge AI allowed emergency teams to act quickly during critical situations, reducing response times and potentially saving lives.
Future-Proofed Infrastructure: The scalable and modular nature of the solution ensured it could evolve with the city’s needs, supporting a growing population and increasing urban demands without requiring significant reinvestment.
Cost Efficiency: Localized data processing reduced reliance on cloud services, resulting in lower operational costs while maintaining system performance. This made the solution more sustainable and economically viable for the long term.
Strengthened Public Trust: With robust privacy protections in place, the system addressed public concerns about surveillance, fostering greater acceptance and trust in the technology.