Product Engineering
Healthcare IoT Company Resolves Latency in Patient Monitoring
Client Background:
The client is a healthcare IoT company specializing in advanced patient monitoring solutions widely used in hospitals, clinics, and homecare environments. Their systems track vital metrics such as heart rate, oxygen levels, and temperature, playing a critical role in ensuring patient safety and enabling timely medical decisions.
As their customer base grew and data volumes surged, they faced significant challenges in maintaining system responsiveness and reliability.
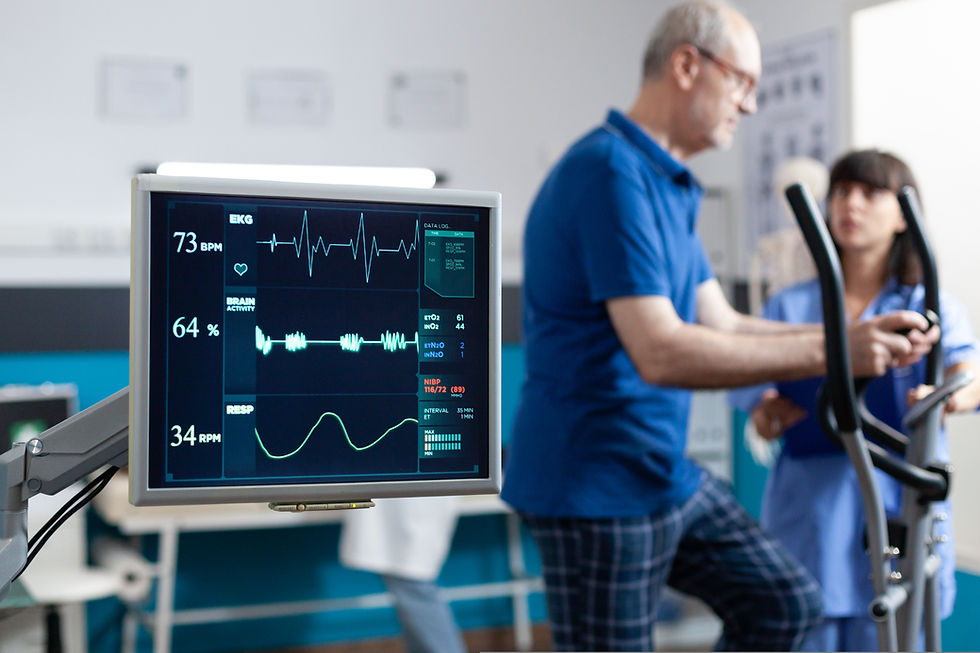
Challenges:
The client's cloud-based architecture could not handle the increasing volume of real-time health data generated by their monitoring devices. Centralized data processing led to delays in transmitting critical information, causing latency issues that jeopardized the timely delivery of health alerts. This latency created risks in emergencies, such as irregular heart rhythms or sudden oxygen level drops, where immediate action was essential. Moreover, the inability to scale the system efficiently with growing data flow constrained their ability to expand services to new healthcare facilities.
The client required a solution to process large volumes of data in real-time, eliminate latency, and ensure future scalability while maintaining security and performance standards.
Our Solutions:
To resolve the latency issues and ensure scalability, we implemented a tailored solution combining edge computing and distributed system architecture.
Edge Computing Implementation: Edge devices were deployed to process and analyze data locally, reducing dependency on centralized cloud servers and minimizing delays in data transfer.
Real-Time Data Flow Optimization: Redesigned data pipelines facilitated faster, more secure communication between devices and systems, ensuring uninterrupted real-time data transmission.
Load Balancing Systems: Integrated load balancing distributed data processing tasks across edge and cloud nodes, preventing system overloads and maintaining consistent performance.
Enhanced System Architecture: Upgraded infrastructure with a hybrid edge-cloud model enabled faster synchronization of data and long-term storage for advanced analytics.
Scalability Enhancements: A modular architecture was designed to accommodate the client’s growth, enabling the system to handle increasing data volumes seamlessly.
Outcomes:
The implemented solution transformed the client’s patient monitoring systems, delivering notable improvements in performance, reliability, and scalability.
Real-Time Health Alerts: Latency was eliminated, enabling the rapid delivery of critical health updates. This ensured that healthcare providers could act immediately in response to any concerning changes in patient conditions.
Data Reliability: The upgraded system guaranteed accurate and timely processing of patient health metrics, building trust among medical professionals who relied on the data for decision-making.
Operational Efficiency: By offloading significant processing tasks to the edge, server dependency was reduced, leading to better resource utilization and cost efficiency.
Scalable Systems: The future-ready infrastructure allowed the client to expand their services seamlessly, accommodating new devices and users without compromising performance.
Improved Patient Outcomes: Faster and more reliable patient monitoring empowered healthcare professionals to respond to emergencies promptly, enhancing the quality of care and saving lives.