Emerging Technology
AI-Powered Edge Computing for Industrial Automation
Client Background:
The client, specializes in industrial automation offers cutting-edge solutions for manufacturing, logistics, and process control sectors. With decades of experience, they assist a wide variety of clientele in increasing operational dependability, decreasing downtime, and optimizing production efficiency. They are continuously searching for novel technologies to keep ahead as market dynamics change.
Their objective was to strengthen their edge computing infrastructure by using AI to speed up data processing, improve automation processes, and provide real-time insights that improve outcomes and decision-making.
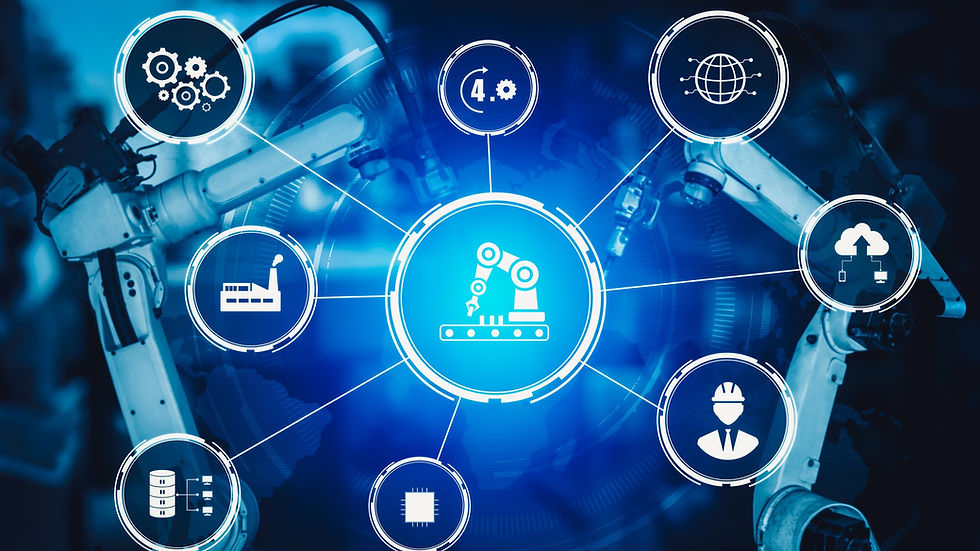
Challenges:
In an industry where every second counts, our client was confronted with several operational hurdles. Their traditional computing infrastructure was struggling to keep pace with the growing volume of real-time data generated by industrial machinery, sensors, and IoT devices. Processing this data centrally created significant delays, which hindered their ability to make timely decisions and impacted overall production efficiency. Furthermore, with their increasing reliance on advanced analytics and AI, the client needed a way to run machine learning models closer to the source to reduce latency and improve predictive accuracy.
Finally, as their global operations expanded, the need for better integration between systems, devices, and locations became critical to maintaining operational coherence and maximizing automation potential.
Our Solutions:
We redefined the client’s industrial automation processes by integrating AI-powered edge computing, creating a more responsive, scalable, and efficient infrastructure.
AI-Powered Edge Analytics: We deployed AI models directly at the edge, allowing for real-time data processing without relying on centralized systems. This made critical decisions faster and more autonomous, enhancing overall system responsiveness.
Hybrid Cloud-Edge Infrastructure: We designed a hybrid architecture combining edge computing with cloud capabilities, giving the client the flexibility to scale and manage data both on-site and remotely, allowing them to optimize cost and performance as their operations grow.
Edge Data Optimization and Management: By implementing advanced data management techniques, we enabled the client to process large volumes of sensor data locally, ensuring bandwidth was optimized and reducing the pressure on centralized infrastructure.
Predictive Insights for Proactive Maintenance: Our solution utilized AI-driven predictive analytics to process real-time sensor data, identifying emerging issues before they escalated. This allowed the client to schedule maintenance activities in advance, minimizing unexpected breakdowns and enhancing operational continuity.
Autonomous Decision-Making on the Edge: We empowered their systems to autonomously execute decisions using AI at the edge. This significantly improved agility, reducing reliance on human intervention and allowing systems to self-adjust based on real-time conditions.
Seamless Device & System Integration: To ensure a unified operation, we focused on integrating a diverse range of devices and systems, from sensors to industrial control systems, enabling seamless data communication and enhancing operational coherence across different locations.
Outcomes:
Our AI-powered edge computing solutions led to transformative improvements in the client’s operations.
Faster Decision-Making: Edge-based AI processing enabled rapid responses to data inputs, allowing systems to make informed decisions almost instantaneously, driving greater operational efficiency.
Reduced Machine Downtime: With predictive maintenance capabilities, the client was able to anticipate failures and perform necessary interventions in advance, drastically reducing unplanned downtime and improving overall machine reliability.
Improved System Agility: The autonomous decision-making capabilities at the edge gave the client greater flexibility in responding to changes in production conditions, leading to faster adaptation and smoother operations.
Streamlined Data Management: By processing data locally, the client was able to optimize network bandwidth and reduce the need for central data processing, making their system more efficient and cost-effective.
Enhanced Global Integration: The integration of systems across various plants and locations helped maintain consistent operations, providing a unified view of data and improving decision-making consistency across regions.
Flexible Infrastructure: With a hybrid cloud-edge infrastructure in place, the client was well-positioned for growth, with the ability to scale their automation systems without compromising performance or requiring substantial reinvestment.